The future of modern healthcare network management
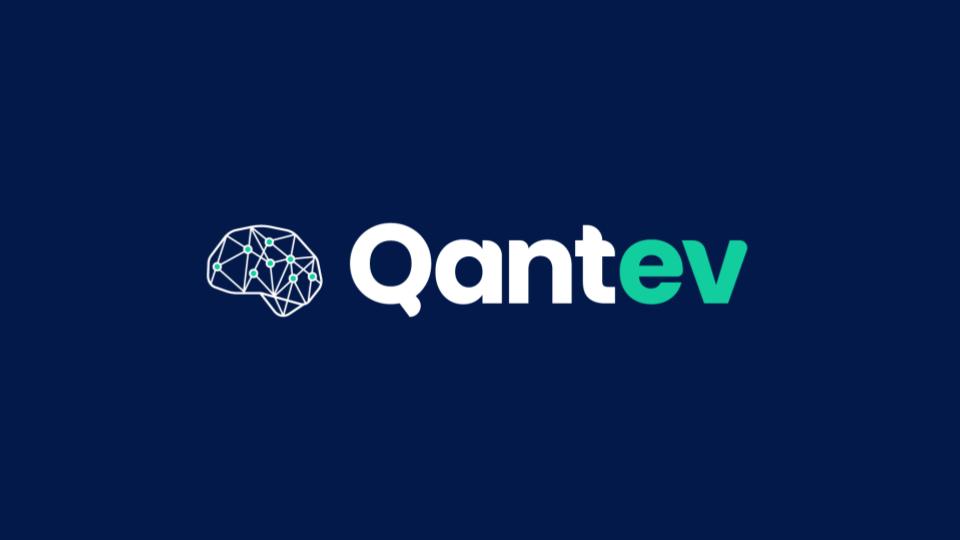
Modern Healthcare Network Management is a critical issue every insurance company should deal with. In order to illustrate this concept, let’s walk through a few use cases that we built with our clients based in the Gulf and Singapore.
A first use case: Provider annual contract renewal
While inflation has been making headlines recently, medical inflation has been significant for decades and makes health care affordability particularly challenging. Medical inflation is contributed to by the increasing age of the population and advances in treatment meaning more people are treated and the treatments themselves have become more complex and more expensive. The result is that medical inflation is much higher than general inflation. Against this background, healthcare providers seek to increase their prices to maintain, or increase, their profit margins. Insurers seek to constrain price rises and steer patients to more efficient providers in order to keep premiums affordable and to maintain their profit margin.
At Qantev we are helping insurers to identify cost effective providers. As an example, during a deployment in Singapore we identified that costs in a very popular hospital, for a very common service, were 33% higher than for similar providers. Qantev was able to highlight opportunities and offer advanced benchmarks to build a business case. Using Qantev’s report our partner negotiated a 15% price discount, resulting in a saving of 800,000 dollars. Without the tools Qantev provides, insurers miss negotiation opportunities and spend more money than they need to.
For insurers, provider contract negotiations are a key element in controlling claims cost. However, these negotiations are frequently conducted almost blindly. Often the procurement team of the insurers identifies high level cost increases and acts on them by negotiating small discounts on the whole price list or by attempting to restructure the network without the ability to model the overall cost impact. In addition, the high level analyses performed are based on low quality information because of the difficulty of reconciling the provider’s price list items with the services that have been registered in the claims.
Some basic statistics such as the growth rate of prices are practically impossible to compute without an algorithm taking into account missing data. This is mostly due to two main factors:
- Medical coding inconsistency between services
- Manually entered fields resulting in mistakes and missing data
Comparing a specific healthcare provider with the rest of the market is hard because mapping their service prices with the cost of similar services within other nearby providers is not always possible, as they are very often using custom medical coding or simply describing the diagnosis and the treatment in free text.
Being able to understand that two different names refer to the same service is necessary if you want to compare apples with apples. Since in a lot of markets, these medical codes are not standardised, this mapping is extremely difficult to do manually. This is why Qantev’s analysis utilises Medical Coding Inference, using specialised Natural Language Processing to do it systematically.
We have also created efficient algorithms which are able to extract the maximum amount of information from the unstructured data received by health insurers, going as far as to understanding each line item in a bill. Indeed, many insurance companies enter a very limited amount of the information available in their systems due to the high cost of manual entry. This situation limits the ability to extract insights and to perform advanced analytics downstream.
Another challenge that arises when it comes to comparing apples with apples is grouping the right services together for comparison. Many insurance companies use some basic techniques to map these services together, with an accuracy which hardly reaches 60%. At Qantev, we developed an innovative approach that allows us to build a mapping that is 99% accurate for any provider (more details on this approach will be presented in an upcoming article). This makes us able to compare the prices of the exact same healthcare services, at different levels of granularity.
Thank you to Dr. Gary Bolger and Jonathan Gray for their contribution.