The emergence of data science in insurance
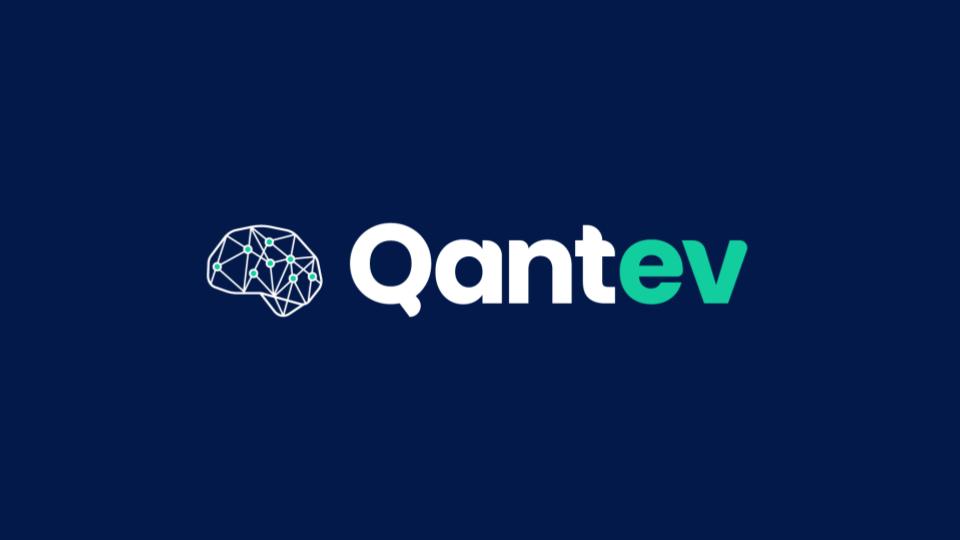
Be it in pricing, provisioning, or risk, predictive models enable insurers to avoid anti-selection, stay competitive while weathering environmental, global health and financial markets hazards.
In this article we will share a partial overview of the current data science insurance landscape and introduce the needs Qantev meets.
On the P&C side, insurance modeling had become increasingly advanced. Examples of this evolution are the finer usage of interpretable boosting models for fair pricing across insurance products and the use of fine-grained geographic information for precise evaluation of systematic risk in property insurance.
The business rules are increasingly being replaced by models in marketing campaigns across the board (cross-selling, upselling and loyalty) as marketing departments aim to improve the relevance of their content to each specific client.
This personalization had been done using traditional statistical models from the Generalized Linear as well as relatively newer Bagging and Boosting models families that became computationally tractable over the last 25 years. Advances on the specification of the mathematical representation had been widespread as well, evolving from propensity models to uplift models, then to a unified Next Best Action approach, grouping outbound activity and aiming to only contact customers on products they need with relevant offers through their preferred channel to improve both customer satisfaction and operations efficiency.
On the healthcare insurance side, one of the emerging analytics topics is Fraud, Waste and Abuse detection (FWA) which are estimated to represent upto 25% of insurance premiums. FWA is getting increasingly important to tackle as medical inflation reaches record levels in many countries and with it, making the healthcare affordability imperative increasingly challenging.
FWA is being addressed with models introduced by emerging data science teams replacing business rules approaches, in order to match the increased sophistication of fraudsters.
On this front, multiple models are used to detect suspicious patterns such as false claims, inflated claims, upcoding, harmful unnecessary surgeries among others, using approaches such as:
- Outlier detection algorithms such as Isolation Forest, LSTM Autoencoder.
- Supervised classification algorithms such as different flavors of Recurrent Neural Networks and Gradient Boosting models.
- Classic statistical tests such as the t-test for cost means equality for cares of the same nature and the Kolmogorov-Smirnov test for equality of distribution for medical codes of comparable medical providers.
Healthcare insurance fills the need to share health risk as a society because the burden of this risk can be crippling. Qantev was born in a context where this field is facing important medical inflation and affordability challenges, while having been underserved so far by analytical tools compared to its P&C counterpart.
In upcoming articles we will further discuss how Qantev enables insurers to improve affordability, coverage and quality of care by designing comprehensive value based networks, tiers and incentives. More specifically we will address how we leverage recent Natural Language Processing breakthroughs along with results from our own research on network optimization.